AI Innovations and Challenges: A Deep Dive into the Latest Developments
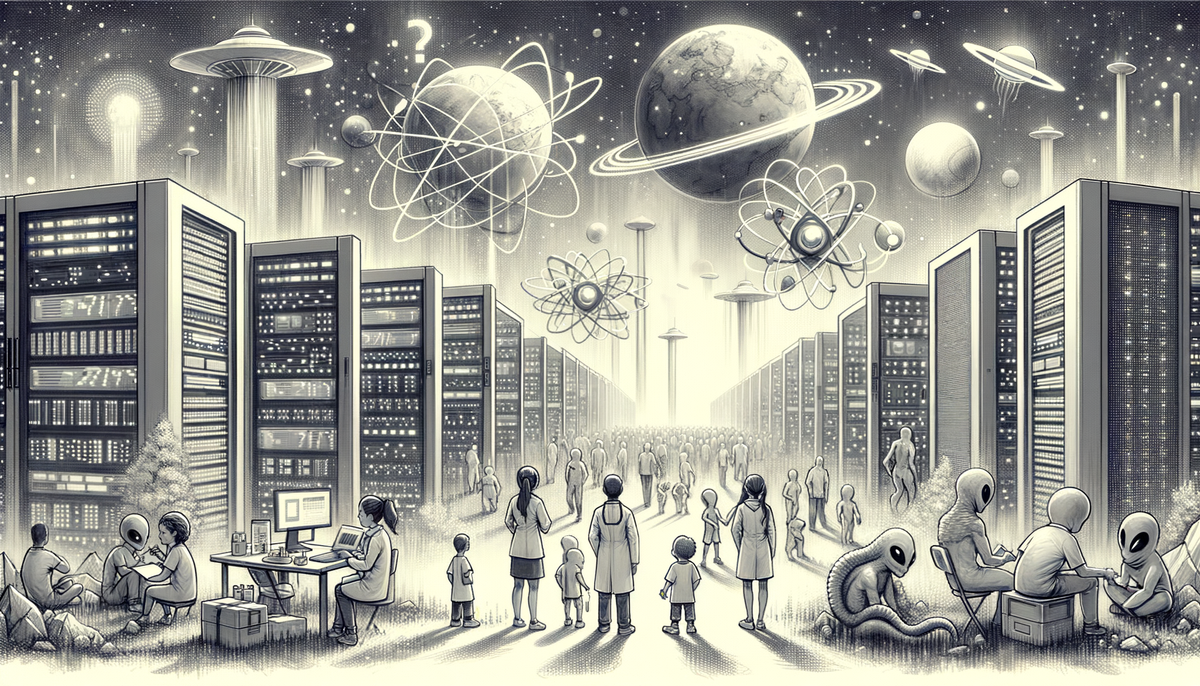
Data centers strained by global supply chain fragilities, AI scrapers challenging the bedrock of free knowledge, and evolving strategies in healthcare and regulatory policy illustrate a landscape in flux—one where advances and constraints coexist in a delicate dance of innovation and caution.
Data Center Constraints: Fueling AI Innovation Amid Supply Chain Challenges
The extraordinary growth in artificial intelligence has sparked a frenetic demand for data centers, a phenomenon that has raised questions about the United States’ ability to maintain leadership in technological innovation. Despite housing 45% of the global data center count, the nation grapples with supply chain obstacles, as key components such as chips and servers are predominantly imported from abroad. This reliance poses risks as nations around the world accelerate investments in domestic chip production. For instance, while the U.S. CHIPS Act of 2022 allocated $280 billion to bolster local manufacturing capabilities, industry analysts warn that the most immediate benefits may not materialize until at least 2028.
Furthermore, rising energy demands compound the strain on existing infrastructure. With projected power consumption skyrocketing to account for 6.6% of U.S. electricity by 2028, regions like Northern Virginia face looming concerns of energy shortages. The situation compels hyperscale operators to explore behind-the-meter power models and adopt renewable energy sources—wind and solar among them—to circumvent potential grid instabilities. Such challenges are a stark reminder that while technological breakthroughs propel us forward, pragmatic strategies and infrastructural investments remain critical to sustaining this rapid pace.
This interplay between supply chain constraints and innovative solutions is discussed in greater detail in our AI Innovations and Challenges series, offering industry insights and pragmatic recommendations for navigating these hurdles.
Guarding Knowledge: The Challenge of AI Data Scrapers
In the digital age, the venerable repository of free knowledge found on Wikipedia is facing an unexpected adversary. As AI developers scramble to gather vast datasets for training their algorithms, automated data scrapers have surged, escalating the load on one of humanity's most cherished knowledge bases. According to recent reports, Wikimedia has experienced a 50% spike in network traffic for image and video downloads since January 2024, primarily driven by these scrapers.
What might appear as a mechanistic data harvest is, in fact, a double-edged sword. While such automated processes accelerate AI learning, they also inflate operational costs for the non-profit foundation that sustains Wikipedia. With higher server demands and potential disruptions to the foundational integrity of an open knowledge resource, the pressure mounts on the Wikimedia community to devise safeguards that balance AI advancement with the ethical use of freely available content.
"Artificial intelligence is the science of making machines do things that would require intelligence if done by men." – John McCarthy
This scenario raises critical questions about how best to ensure that technological progress does not come at the expense of reliable and freely accessible information. In light of this, conversations around data ethics and automated content harvesting are becoming increasingly relevant, urging regulators, organizations, and tech companies to develop sustainable practices that protect public knowledge.
Balancing Innovation and Regulation: Antitrust Perspectives in AI
The rapid evolution of AI brings with it not only technological breakthroughs but also a host of regulatory considerations. In a detailed presentation before the House Antitrust Subcommittee, industry expert Joseph V. Coniglio expounded on the complex relationship between AI and antitrust policies. His remarks centered on three intersections: the competitive dynamics within the AI sector, the broad influence of AI across multiple industries, and its impact on established enforcement practices.
Coniglio argues that, despite rising concerns, the competitive landscape in AI remains robust. Giants like Nvidia, along with disruptive innovators such as OpenAI, have cultivated a dynamic market environment that fuels innovation. This competitive spirit, he contends, could be stifled by adopting overly strict regulatory measures—especially those reminiscent of European models that historically place competitor protection at the forefront. The risk of overly rigid antitrust enforcement could inadvertently slow down the very innovation it seeks to govern.
Moreover, the advent of AI brings with it new challenges for traditional regulatory frameworks. With AI's ability to detect collusion and enhance market monitoring, regulators are now equipped with powerful tools that could reinforce, rather than restrict, competitive practices. As Coniglio emphasized, the guiding principles of the Sherman Act must continue to inspire policies that encourage agility and growth. It is the delicate equilibrium between regulation and innovation that will ultimately determine the trajectory of the AI revolution.
Advancements in Healthcare: Gen AI and AI-Driven Clinical Decisions
Healthcare, one of the most intricate and sensitive sectors, is witnessing a transformation powered by generative AI (Gen AI). According to a recent McKinsey & Company survey, an impressive 85% of healthcare leaders are either exploring or actively implementing Gen AI technologies. The primary objective has been to streamline administrative processes, yet the ripple effects of these innovations extend to clinical productivity and patient engagement.
Collaborations with third-party vendors, notably with established IT firms and hyperscalers, signal that healthcare organizations are not only embracing AI for efficiency gains but are also laying the groundwork for more robust data management systems. The ability of these implementations to generate measurable returns on investment (with 64% of respondents reporting positive ROIs) reinforces the potential of AI to deliver significant value in a traditionally conservative industry.
In parallel, groundbreaking research in urgent care settings has unveiled that AI-driven treatment recommendations can surpass human clinical judgment. In a study presented at the American College of Physicians meeting, AI models demonstrated optimal treatment quality in over 77% of cases compared to 67% for seasoned clinicians. These findings are shifting paradigms in medical decision-making and are prompting deeper discussions about the future role of AI in clinical environments.
While these advances herald a promising future, they also necessitate rigorous oversight—addressing issues like data security and potential algorithmic bias. As we ponder these innovations, it is clear that the integration of AI in healthcare is not just about efficiency, but also about reshaping the fundamental doctor-patient dynamic. The convergence of administrative efficiency and superior clinical decision-making exemplifies the transformative potential of AI-driven tools in healthcare.
Learning Through Play: Google's Dreamer and the Future of AI Self-Improvement
In a captivating illustration of how AI can learn and evolve autonomously, Google's AI project known as “Dreamer” is venturing into the imaginative realms of Minecraft. Leveraging reinforcement learning, Dreamer engages with the game environment to master complex tasks, honing its decision-making skills through trial and error—a process reminiscent of how children learn through play.
In this virtual sandbox, Dreamer receives reinforcement signals that help it identify effective strategies. When it successfully completes tasks like constructing a shelter or efficiently gathering resources, the algorithm is rewarded, embedding those successful behaviors for future challenges. This dynamic cycles of learning and self-improvement hint at broader applications. Whether in robotics or complex simulation environments, the principles distilled from gaming can be harnessed to solve real-world problems.
This approach not only redefines the boundaries of traditional machine learning but also underscores the growing trend of using engaging digital platforms to accelerate AI development. Dreamer’s journey through Minecraft is a microcosm of how technology can employ unconventional training methods to foster resilience and adaptability in machines, taking cues from environments that spark creativity and innovation.
Evolving User-Centric AI: Shifting Strategies at OpenAI
In the ever-competitive world of conversational AI, OpenAI has recently decided to put the much-anticipated ChatGPT-5 on hold—a move that reflects the company's nurturing approach to technological evolution. Instead of rushing a release, OpenAI is channeling its efforts into refining the new o3 and o4-mini models. This pivot comes in the wake of unprecedented user demand, which has recently led to system outages and frustration among its expansive user base.
The new models, set for imminent launch, signify a deliberate shift toward enhancing user interaction and performance. OpenAI envisions these iterations as having the dual capability to intelligently determine whether a user should engage with a lighter model or a more robust one. This degree of personalization holds the potential to refine the user experience substantially. Additionally, the company is taking strides to ensure that its AI offerings remain broadly accessible, extending benefits even to free-tier users while reserving advanced features for higher-end subscription models.
This strategic decision underscores a broader industry trend: the necessity to balance rapid innovation with sustainable operational practices. Instead of succumbing to external pressures, OpenAI is choosing a measured approach—one that prioritizes long-term efficacy over short-term gains. Such thoughtful recalibrations echo the sentiment that strategic pauses are sometimes essential for paving the way toward more robust, user-centric technological ecosystems.
The Complexities of AI in Government: Innovations and Cautions
Government initiatives in the realm of AI carry a unique weight—balancing the promise of technological innovation with the responsibility of serving millions. At the Department of Veterans Affairs (VA), a particularly contentious scenario has unfolded, spearheaded by operatives from Elon Musk's DOGE team. Under the advisement of Sahil Lavingia, a relatively inexperienced startup CEO, transformative yet controversial proposals have emerged to integrate AI into federal systems.
Lavingia's proposals, including the use of AI-powered tools to pre-fill forms and automate coding processes, are intended to revolutionize service delivery at the VA. However, such sweeping changes have raised valid concerns among employees and congressional representatives alike. The potential risks—ranging from the inadvertent introduction of software vulnerabilities to the undermining of traditional safeguards—underscore the challenges of implementing cutting-edge technology within a high-stakes government setting.
While the promise of increased efficiency and digitization is alluring, critics caution against precipitous changes that might compromise the quality of essential services for the nation’s veterans. The VA’s struggle to balance innovation with security highlights the perennial tension between rapid technological upgrade and the need for rigorous oversight. As the public sector ventures further into AI integration, establishing robust ethical frameworks and protective measures remains paramount.
Integrating Lessons Across Sectors: The Road Ahead for AI
Reflecting on the multifaceted developments across industries, it becomes evident that artificial intelligence is not merely a tool for automation—it is a transformative force that reshapes the very foundations of modern society. From the infrastructural demands of data centers and the ethical dilemmas of content scraping to the disruptive potentials within healthcare and government services, AI is challenging conventional wisdom at every turn.
The journey of AI, illustrated by research findings and practical implementations alike, reminds us of the timeless truth articulated by Fei-Fei Li: "If our era is the next Industrial Revolution, as many claim, AI is surely one of its driving forces." This sentiment resonates across sectors and underscores a critical lesson: innovation must be pursued with an unwavering commitment to adaptability, ethical responsibility, and strategic foresight.
Our ongoing coverage at AI.Biz—exploring topics such as digital assistance innovations and the evolving landscape of AI developments—offers deeper insights into how these trends interweave. Together, these narratives shape a comprehensive picture of the challenges and triumphs in our rapidly evolving digital world.
In conclusion, the AI journey is a tapestry woven with threads of technological ambition, infrastructural challenges, and ethical quandaries. As we navigate this complex terrain, it is both exciting and essential to continue engaging in informed dialogue and vigilant innovation, ensuring that as we propel into the future, we do so with our eyes wide open to both promise and peril.
Further Readings
- Addressing Data Center Growth Constraints Key to U.S. Innovation, Leadership in AI – POWER Magazine
- AI Data Scrapers: An Existential Threat to Wikipedia – New Scientist
- Antitrust and AI: Remarks Before the House Antitrust Subcommittee – ITIF
- Survey: Gen AI in Healthcare Gains Momentum Across Sectors – TechTarget
- AI Bests Clinicians in Urgent Care Treatment Recommendations – HCPLive
- Google's AI Dreamer Learns How to Self-Improve Over Time by Mastering Minecraft – Tech Xplore
- ChatGPT-5 on Hold as OpenAI Shifts Gears – TechRadar
- Inside DOGE’s AI Push at the Department of Veterans Affairs – Wired
In the vibrant crossroads of technological potential and real-world constraints, one truth rings clear: our future with AI is being written today, blending bold visions with careful stewardship—an evolution that continues to surprise and inspire.