AI Training Paradigms: Innovation, Challenges, and Future Directions
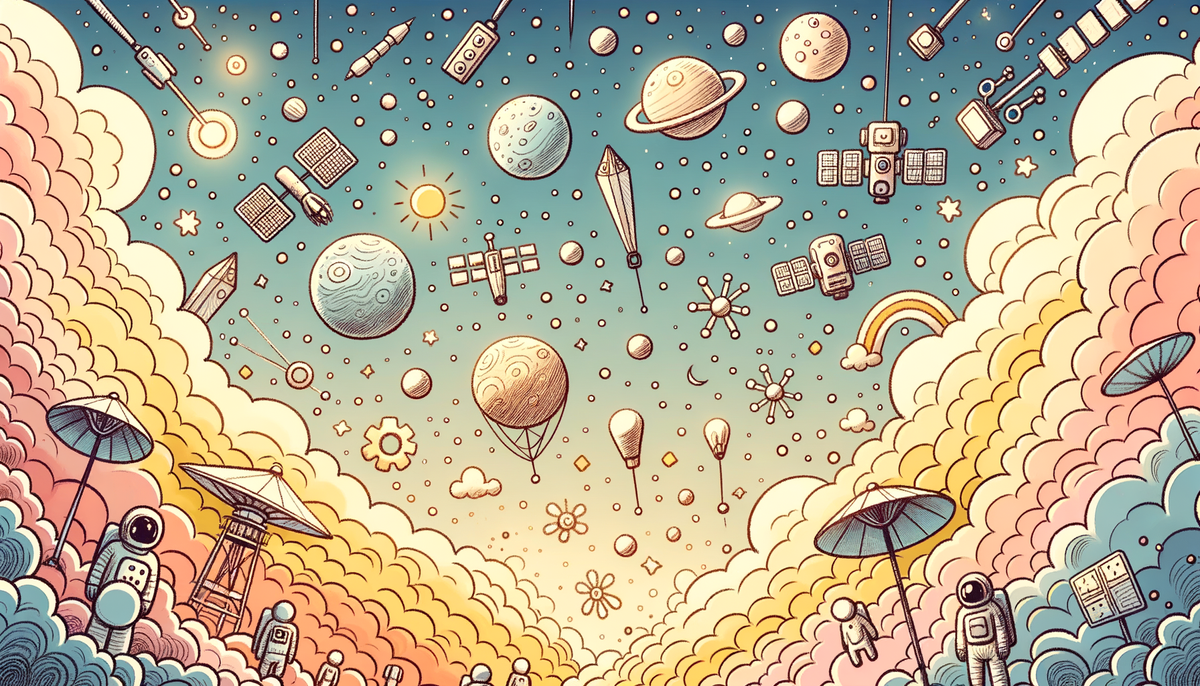
Apple’s candid confession of “ugly and embarrassing” delays in Siri’s evolution sets a dramatic tone in the AI arena where innovation battles bureaucracy, licensing hurdles, and mounting project failures across the industry.
Struggles in Voice Assistance: Siri’s Uphill Battle
During a recent all-hands meeting, Apple’s Senior Director of Siri and Information Intelligence, Robby Walker, openly described the delays of crucial Siri features as “ugly and embarrassing.” This blunt admission reveals that while competitors are racing ahead in enhancing virtual assistants, Apple finds itself beset by internal challenges and shifting priorities. The much-anticipated on-screen awareness and personal context enhancements have been pushed back to at least 2026, fueling skepticism about Siri’s capability to reclaim a leadership position in a market where convenience and accuracy dictate dominance.
The sigh of disillusionment is palpable within the tech community, as even loyal users wonder if a complete overhaul might be necessary for Siri to truly compete. The issues extend beyond simple functionality improvements; they point to deeper systemic difficulties in balancing innovation with operational pressures. In a landscape where voice assistants are increasingly intertwined with daily digital interactions, any hesitation risks sidelining an essential technology.
Reflecting on these struggles, it becomes clear that this isn’t an isolated incident. Similar challenges surface across various AI initiatives, highlighting the necessity of agile project management and flexible innovation strategies. For further perspectives on similar challenges and industry responses, consider exploring our discussion on Google’s Shift to Gemini, which also delves into transformative yet challenging transitions in digital assistant technologies.
Licensing Labyrinths and Open Model Restrictions
In parallel to Siri’s internal struggles, the licensing landscape for AI models remains fraught with uncertainty. Google’s recent emergence of the Gemma 3 models has been met with applause for efficiency, but it comes shadowed by the restrictive licensing terms provided with these models. This cautious approach to open model distribution isn’t an isolated strategy; Meta’s Llama models are similarly hampered by restrictive contractual arrangements. Developers and businesses face potential legal pitfalls from sudden policy shifts and ambiguities in the terms of use.
These custom open-source licenses, as critiqued by voices in the developer community, stifle creativity rather than foster collaborative innovation. The Open Source Initiative has cautioned that such convoluted terms create an unsettling environment for smaller firms that lack the legal expertise to navigate this turbulent legal terrain. Consequently, many are gravitating toward more transparent and established models such as the Apache 2.0 license. This sentiment echoes a broader warning: without clear and unified licensing standards, the rapid pace of AI development may be hampered by legal disputes and operational uncertainties.
"AI is likely to be either the best or worst thing to happen to humanity." – Stephen Hawking, Brief Answers to the Big Questions
While innovators push the boundaries of what AI can achieve, licensing restrictions serve as a sobering reminder of the complex interplay between technology and regulatory frameworks. For a closer look at the intricacies of how such legal constraints are shaping AI policy debates, readers might find our piece on OpenAI and Google’s Policy Initiatives on Training AI to be particularly illuminating.
The Battle Over Copyright: Training Data and Innovation
AI development does not exist in a vacuum; it is enmeshed with broader legal and ethical concerns, notably around the use of copyrighted material. OpenAI and Google have taken a bold step by appealing to the U.S. government for permission to use copyrighted content in training their AI systems. Their argument centers on the need to harness vast amounts of data for cutting-edge AI research and development, particularly in a climate where international competition—especially from China—is fierce.
The technological imperative to gather expansive datasets collides with strict copyright laws, compelling regulators to re-evaluate the "fair use" doctrine under current policies. By invoking exceptions for data mining and fair use, both companies are attempting to sidestep potential legal roadblocks that could encumber the pace of AI innovation. Yet, this approach remains contentious, drawing scrutiny from various stakeholders who worry that too lenient an interpretation might undermine creators’ rights.
Interestingly, not all industry players echo the same concerns. Anthropic, for instance, has pivoted its focus towards assessing national security risks rather than wrestling with copyright disputes. This divergent approach underlines the multifaceted nature of AI challenges, where legal, ethical, and national security considerations intermingle to influence decision-making at the highest levels.
For businesses and policymakers alike, this debate serves as both a warning and an opportunity. By rethinking the legal parameters of AI training, there may be a path to fostering an environment where innovation is not held hostage by outdated copyright constraints. More insights on the evolving regulatory landscape can be found in our earlier update at this link.
Recent Trends: Rising AI Project Failure Rates
Amidst breakthroughs and buzz, recent data reveals a stark and concerning trend: AI project failure rates are spiking. A report by S&P Global Market Intelligence indicates that the proportion of companies abandoning their AI projects has surged, with an alarming 42% tipping the scales this year compared to 17% in 2024. Nearly half of pilot projects fail to transition into production—a sobering statistic that reflects the inherent challenges of integrating AI into complex business processes.
Cost overruns, data privacy concerns, and technological missteps contribute significantly to project discontinuation. What might seem like failures are often integral to fostering an environment of experimentation, where missteps pave the way for eventual breakthroughs. Companies that pivot quickly, analyze lessons learned, and pivot their strategies tend to succeed, even in the face of mounting obstacles. Celebrating such learning experiences can promote a culture of resilient innovation rather than an atmosphere of stifled creativity and fear of failure.
Developers and business leaders are rethinking their strategies, acknowledging that a measured approach—where the technology is carefully integrated to meet specific needs—is paramount. This recalibration may lead to more focused applications of AI, ensuring that initiatives are both sustainable and scalable over time. For an expanded study on AI project dynamics, you might also want to explore our in-depth discussion on AI Project Failure Rates, which offers further analysis of the economic and organizational challenges in AI adoption.
Healthcare Innovated: Nimblemind.ai’s Data Revolution
While some areas of AI falter under bureaucratic and legal pressures, others are thriving by addressing underserved challenges. Nimblemind.ai is a prime example of using AI to solve critical issues in healthcare by transforming messy, unstructured patient data into actionable insights. With a recent funding injection of $2.5 million led by Bread & Butter Ventures, the company is poised to revolutionize how healthcare institutions harness AI for predictive analytics and personalized treatment.
At the heart of Nimblemind.ai’s platform is a commitment to quality data curation. CEO Pi Zonooz emphasizes that without meticulously structured and labeled clinical information, the promise of AI in healthcare remains largely untapped. By forging strategic partnerships across both the US and Asia, Nimblemind.ai is building a robust network that ensures data integrity and drives improved patient outcomes.
This advancement in healthcare technology is emblematic of how AI can transcend its typical narratives of automation and efficiency, reaching into the realms of personalized medicine to make a tangible impact. The healthcare industry, known for its conservativism and stringent regulatory requirements, now stands on the cusp of a data-driven revolution. As security, privacy, and reliability remain critical, platforms like Nimblemind.ai offer a glimpse into the future of healthcare where cutting-edge AI informs every clinical decision.
The success of such initiatives invites comparisons to other AI breakthroughs highlighted elsewhere. It underscores the fact that while some sectors wrestle with ethical and legal concerns, others are forging ahead by solving practical, day-to-day problems with innovative technology. This dichotomy exemplifies the multi-dimensional nature of AI’s role in modern society.
The Evolution of Digital Assistants: Google’s Gemini Takes Over
In an era defined by rapid technological evolution, even the stalwarts of digital assistance are not immune to reinvention. Google’s recent decision to replace its long-standing Google Assistant with the next-generation Gemini signals a major paradigm shift in how we interact with technology. Slated to roll out across Android devices later this year, Gemini promises a suite of enhanced functionalities—from seamless music playback to intuitive actions available directly from lock screens.
This bold transition is indicative of a broader trend in the industry: the constant quest to deliver more intuitive, context-aware, and responsive digital experiences. Google’s confidence in Gemini is a testament to its commitment to innovation, even as it phasing out a product that, for many users, has been a trusted companion. The transition is being managed with care, ensuring continuity for current users even as cutting-edge features are gradually introduced.
Moreover, this change reflects a strategic response to stiff competition, especially in a market where voice and digital assistants are increasingly intertwined with personal and professional routines. Readers interested in the evolving trends of digital assistance might enjoy reading our detailed piece on Google’s Shift to Gemini, which provides further context on how emerging technologies are redefining user interaction paradigms.
Nvidia’s Pivot: Beyond Chips to Holistic AI Innovations
Nvidia’s journey from a chip manufacturer to a multitrillion-dollar force in AI is a narrative defined by bold gambles and rapid reinvention. Recently, CEO Jensen Huang signaled an expansion of Nvidia’s AI ambitions that goes beyond its core business of producing high-performance chips. With threats of market saturation, increasing competition, and geopolitical challenges, Nvidia is now charting a course toward integrated AI solutions across multiple sectors.
The move underscores a recognition that true AI innovation requires more than just raw hardware power; it calls for sophisticated software, innovative platforms, and a holistic approach that can weave AI into everyday operations. Nvidia’s ambition to explore advanced AI agents and even physical AI implementations—ranging from robotics to autonomous systems—marks a turning point in the industry. The challenges are formidable, with market fluctuations and headwinds from international trade dynamics, yet Nvidia’s proactive strategy positions it well for the future.
This pivot reflects a broader industry trend observed in other parts of the AI ecosystem. As companies like OpenAI and Google strive to navigate regulatory landscapes and optimize their training methodologies, traditional hardware leaders are forced to think beyond their established domains. The integration of software, services, and hardware into a cohesive ecosystem isn't just a business strategy—it’s a reflection of how AI is becoming omnipresent in our interconnected world.
For additional insights into Nvidia’s strategic moves and how they fit into the larger narrative of AI progress, you can check out our earlier coverage of Nvidia’s expansion efforts at PYMNTS.com, which delves into the industry challenges and opportunities from this giant’s perspective.
Intertwining the Threads: A Multifaceted AI Landscape
The interviews, open letters, and internal memos circulating in today’s AI narrative reveal an industry at a crossroads. On one hand, there’s the painful reality of product delays and internal struggles as seen in Apple’s tardy rollout of next-generation Siri. On the other, the tension between legal frameworks and technological innovation continues to challenge leaders like OpenAI and Google when it comes to utilizing external data sources for training advanced models.
Diverse aspects—from the restrictive open model licenses hindering creative use to a surge in project failures stemming from budget and privacy concerns—paint a picture of an industry that is as challenged as it is promising. Amid these trials, there are also inspiring success stories such as Nimblemind.ai, which is remolding the landscape of healthcare with transformative data strategies.
What emerges is a tapestry of strategies that defy a simple narrative of technological triumph or failure. Instead, the AI story unfolds as one of resilient innovation, constant iteration, and, above all, adaptation to an ever-changing global environment. Whether it’s through insightful policy debates, rigorous rethinking of open-source licensing norms, or radical reinventions like Google’s Gemini, the very fabric of AI is woven from bold ideas and measured risks.
Reflecting on this multifaceted landscape, I am reminded of Larry Page's insightful remark: "We will be able to use machines to augment human intelligence, but never to replace it. The creativity of the human mind will always be the final frontier." This sentiment resonates deeply when considering the overarching ambitions of AI initiatives—from voice assistants trying to recapture lost ground, to enterprises pivoting their entire strategy in response to the unpredictable challenges of AI research and development.
In this era where the theoretical meets the practical, the interplay between innovation and regulation is more significant than ever. It is a dynamic interplay that will shape not only how AI technology develops, but also how society as a whole incorporates these advancements into everyday life.
Looking Forward: Embracing a Culture of Resilient Innovation
Every setback in the AI world carries the seed of future innovation. The painful acknowledgment of delays in improving Siri, for example, may provide Apple a crucial opportunity to reimagine digital assistance from the ground up. Meanwhile, the challenges posed by restrictive licensing and copyright debates compel industry leaders to rethink the very frameworks that support AI research—a necessary evolution that may very well unlock new platforms of cooperation and creativity.
In a climate where AI project failures make headlines as cautionary tales, it is important to remember that experimentation is a cornerstone of technological progress. Embracing failure, learning from missteps, and iterating on feedback are practices just as vital as breakthroughs themselves. This philosophy is not confined to Silicon Valley boardrooms alone; it’s evident everywhere—from nimble startups like Nimblemind.ai revolutionizing healthcare to tech giants like Nvidia redefining their corporate boundaries.
The road ahead is undoubtedly filled with regulatory hurdles, operational challenges, and fierce competition. However, these hurdles also present an invitation to innovate relentlessly. As we continue to witness paradigm shifts—from AI’s integration into everyday devices to the audacious moves of major corporations navigating global markets—the collective experience of the industry offers a promising signpost: the future of AI is bright, adaptable, and ultimately defined by the willingness to take calculated risks.
If you’re keen on exploring more about these transformative trends, our articles such as Why Gen AI and Cybersecurity Are Foundational further examine how these shifts impact industries like education and beyond.
Further Readings
- OpenAI and Google Seek Freedom to Train AI on Unauthorized Content
- AI Project Failure Rates Are On The Rise: A Wake-Up Call for Innovation
- Google’s Shift to Gemini and the Evolving AI Landscape
- Google Replaces Google Assistant with Gemini: A New Era for Digital Assistants
- Apple’s Internal Report on Siri Delays
- ’Open’ Model Licenses and Their Concerning Restrictions
- The Copyright Conundrum: OpenAI and Google’s Bold Appeal
- Nimblemind.ai Raises $2.5 Million in Healthcare AI Innovation
- Nvidia Expands AI Efforts Beyond Chips