Humans overestimated Space Travel, Flying Cars, Clean Nuclear Energy. Are we doing that with AI too?
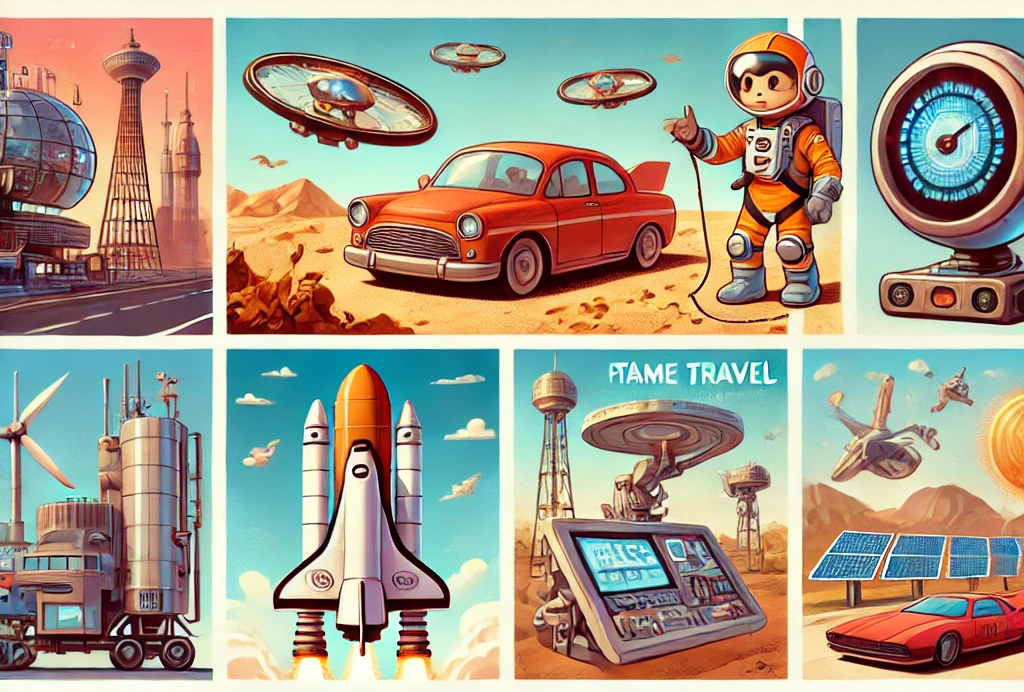
The hype is everywhere. The investments are massive. But what if we’re expecting too much, too soon?
We’ve been here before: flying cars, Mars colonies, time travel, limitless nuclear energy. Each a beautiful dream—each brought down to earth by complexity.
“History warns us—we’ve overestimated before. Flying cars, Mars colonies, time travel, limitless nuclear energy. Beautiful ideas, all stopped by practical walls. Complexity often outweighs fantasy.” — Sameer Gupta
AI is no different. We’re approaching a point where the cost of improvement is outweighing the benefit. GPT-3 changed the world for under $200 million. GPT-4 cost more than $1 billion—and the leap was smaller. GPT-5 might cost $10 billion. Will it be 10 times smarter? Doubtful.
“The law of diminishing returns will soon slow AI’s progress unless quantum computing arrives. No one wants to invest 10x for just a 10% gain in intelligence.” — Sameer Gupta
Meanwhile, hardware isn’t keeping up. GPUs are expensive. Power-hungry. And they’re approaching the limits of what’s physically possible.
“Like CPUs before them, GPUs too will eventually hit physical limits. Moore’s Law may bend, but nature still sets the rules.” — Sameer Gupta
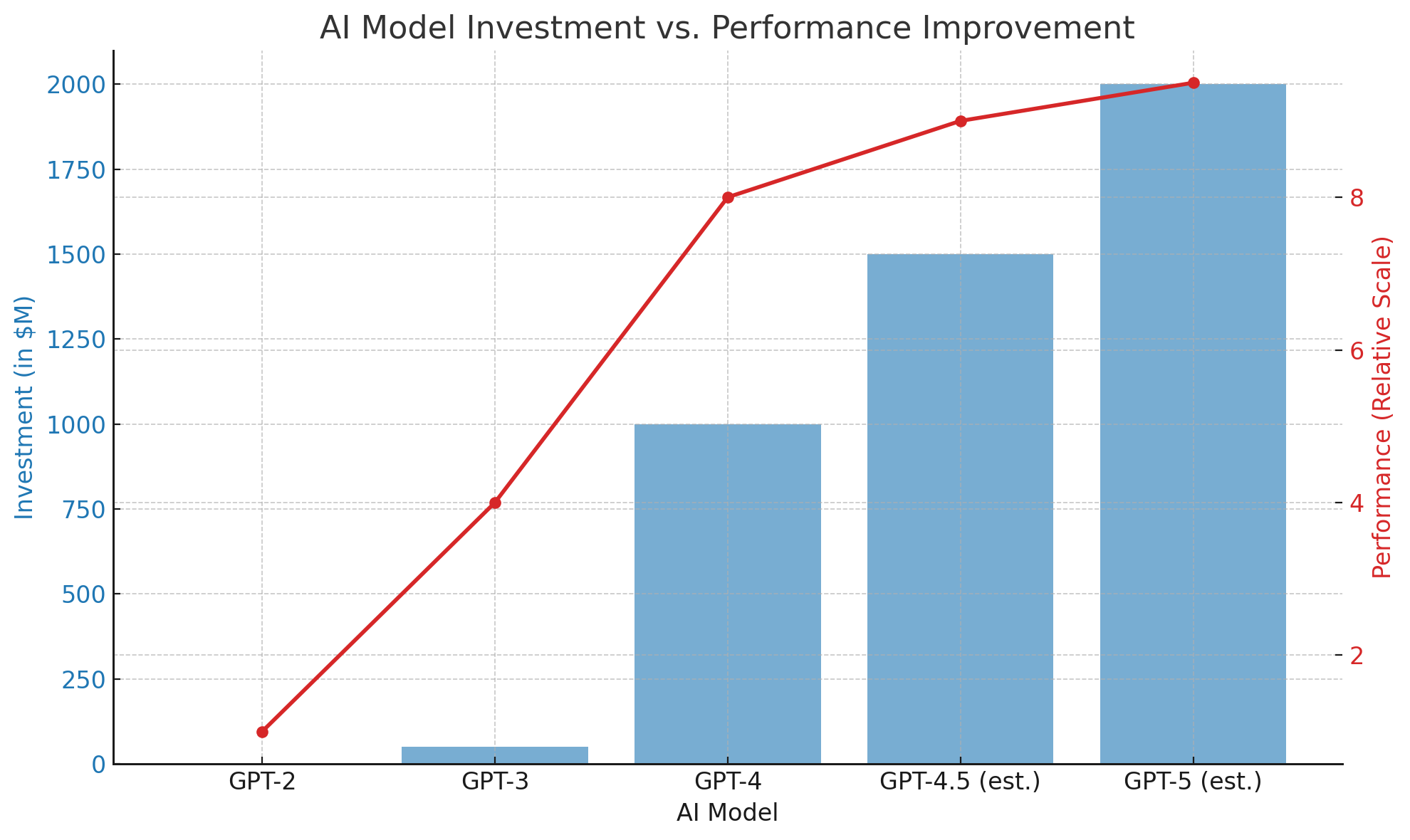
The NVIDIA Strategy: Can It Hold?
To stay ahead of these limits, NVIDIA has innovated beyond just chip design. The company now focuses on optimizing the entire computing stack—from hardware architecture to systems integration, and even to software frameworks and AI models themselves. This full-stack optimization has allowed NVIDIA to continue delivering performance gains even as traditional transistor scaling slows.
But can this approach scale indefinitely?
Software optimization has diminishing returns too. Eventually, we’ll reach a point where every instruction is tightly packed, every layer fine-tuned, every pipeline reworked. Once that ceiling is hit, even the most cleverly optimized stack won’t deliver major breakthroughs unless something fundamentally new—like quantum computing, neuromorphic chips, or breakthrough materials—comes into play.
In other words, even the best engineering teams can only buy us time.
"Moore's Law was about transistor counts. NVIDIA’s strategy is about entire systems. But both face the same enemy: physical limits." — Sameer Gupta
What's Next? A Glimpse at Frontier Technologies
Quantum Computing
Quantum computing operates on principles of quantum mechanics—using qubits instead of bits. Qubits can exist in multiple states simultaneously, enabling them to perform certain computations exponentially faster than classical computers.
For AI, quantum computing holds the promise of accelerating training, solving optimization problems faster, and modeling complex systems that are intractable today. But it’s still in early stages. Companies like IBM, Google, and startups like Rigetti and IonQ are racing to improve qubit coherence, error correction, and scalability. It may take 5–10 more years before quantum processors become truly viable for mainstream AI applications.
Neuromorphic Chips
Neuromorphic computing mimics the brain’s structure and function using spiking neural networks and event-driven processing. These chips process data in a fundamentally different way—prioritizing energy efficiency and real-time response over brute force.
Intel's Loihi, IBM’s TrueNorth, and startups like BrainChip are experimenting with neuromorphic systems that promise ultra-low power consumption, better edge computing, and faster decision-making for robotics, autonomous systems, and sensory data processing.
While these systems may not replace GPUs for large model training, they could revolutionize how AI is deployed—particularly in embedded and real-time environments.
Breakthrough Materials
Silicon has taken us far, but it has limits. Researchers are now exploring new materials:
- Graphene: a superconductor with extremely high electron mobility.
- Gallium Nitride (GaN): already used in some high-power devices.
- Carbon Nanotubes: potential for miniaturization far beyond silicon.
These materials may help reduce heat, shrink transistors further, or create entirely new chip architectures. However, transitioning from lab experiments to mass production is a massive hurdle that can take decades.
Are We Overestimating AI?
Maybe. But not in its impact; in its pace. The transformation is coming. But it won’t be a Hollywood moment. It’ll be quiet, steady, everywhere. Already, AI is transforming agriculture, healthcare, translation, logistics, and education.
The real opportunity isn’t to build the smartest model. It’s to build the most useful one.
Because when AI does something well—no one goes back.
Final Thought
We don’t need to stop building. But we do need to shift focus—from more horsepower to smarter engineering. From headline-grabbing models to real-world applications.
“What AI can do, it will eventually do. And what we can use it for, we inevitably will.” — Sameer Gupta
Let’s not overestimate what comes next. Let’s build better with what we have now—and prepare for what’s still just over the horizon.